Hey there, future data wizards! Welcome to Statssy! Ever wondered what makes research not just good, but amazing? The answer is simple: Data Analysis.
Data analysis is like the secret sauce that takes your research from “meh” to “mind-blowing”! It’s all about sifting through heaps of data to find those golden nuggets of wisdom.
Why is it a Big Deal?
Well, data analysis helps you make sense of all the information you’ve gathered. It’s the bridge between collecting data and actually using it to make informed decisions.
Show Me the Numbers!
Don’t just take my word for it. Check out this pie chart that shows how researchers feel about the importance of data analysis in their work.
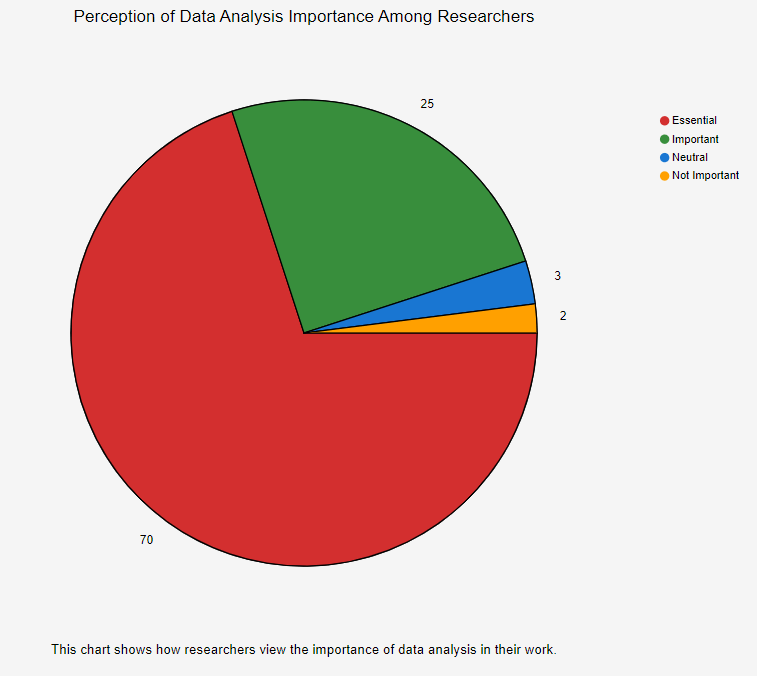
As you can see, a whopping 70% of researchers consider data analysis to be essential. That’s a lot of love for data, right?
So, are you ready to dive into the world of data analysis? Let’s go!
Why is Data Analysis in Research Important?
Alright, so you’re probably thinking, “Cool, data analysis sounds fun, but why should I care?” Well, let me break it down for you!
The Role of Data Analysis
Imagine you’re a detective, and you’ve got all these clues (data) scattered around. Data analysis is like piecing together these clues to solve the mystery (your research question). It’s the hero that turns your raw data into something meaningful.
Objectivity and Discovery
Data doesn’t lie, people! Data analysis helps you stay objective by focusing on what the numbers are actually saying, not what you think they should say. Plus, it’s like going on a treasure hunt. You never know what incredible insights you’ll discover along the way!
Show and Tell
Let’s get real for a sec. Check out this stacked bar chart that shows the impact of data analysis on different aspects of research projects.
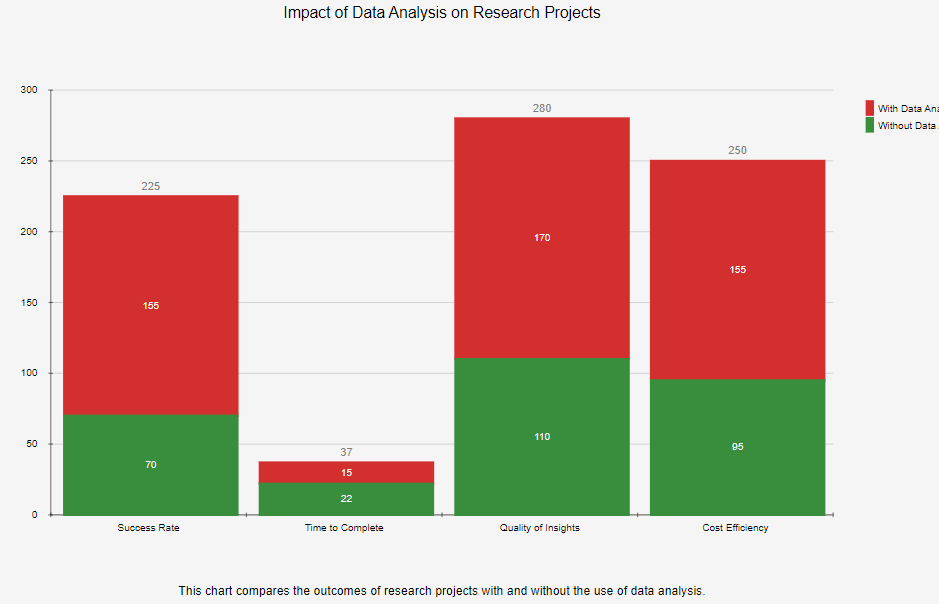
See the difference? Projects with data analysis have higher success rates, better quality insights, and are generally more cost-efficient.
So, if you want your research to be the star of the show, you better not skip the data analysis part!
Qualitative Data
Alright, let’s dive deeper into the world of qualitative data. This is where you get to explore the “why” and “how” of things.
Examples of Qualitative Data
- Interview Transcripts: Imagine you’re researching why Gen Z prefers streaming services over traditional TV. You could conduct interviews and analyze the responses.
- Social Media Posts: You could analyze tweets or Instagram captions to understand public sentiment about a trending topic.
- Open-Ended Survey Responses: When you ask people to describe their experience at a music festival, their answers are qualitative data.
- Field Notes: Anthropologists often take notes during fieldwork. These observations can provide deep insights into human behavior.
- Diaries and Journals: Personal reflections can offer valuable data for psychological or sociological research.
Quantitative Data
Now, let’s talk numbers! Quantitative data is all about measurable information.
Examples of Quantitative Data
- Test Scores: If you’re studying the effectiveness of a new teaching method, you could look at the test scores of students.
- Heart Rate: In a health study, you might measure people’s heart rates under different conditions.
- Temperature Data: Climate researchers often use temperature records to study global warming.
- Sales Figures: Businesses love this! How many units of a product were sold last month?
- Social Media Metrics: Yep, the number of likes, shares, and comments on your posts are all quantitative data.
Categorical Data
Last but not least, let’s talk about categorical data. This is the type of data that falls into specific categories (duh!).
Examples of Categorical Data
- Blood Types: You’re either A, B, AB, or O. There’s no in-between.
- Movie Genres: Whether it’s action, comedy, or drama, each movie falls into a specific genre.
- Academic Grades: A, B, C, D, or F—your grade falls into one of these categories.
- Customer Satisfaction: When you rate a service as excellent, good, average, or poor, that’s categorical data.
- Political Affiliation: Democrat, Republican, Independent—these are categories that people’s political beliefs can fall into.
Which One is Yours?
Still not sure what you’re dealing with? Don’t sweat it! Just refer back to our handy flowchart, and you’ll be a data classification pro in no time.
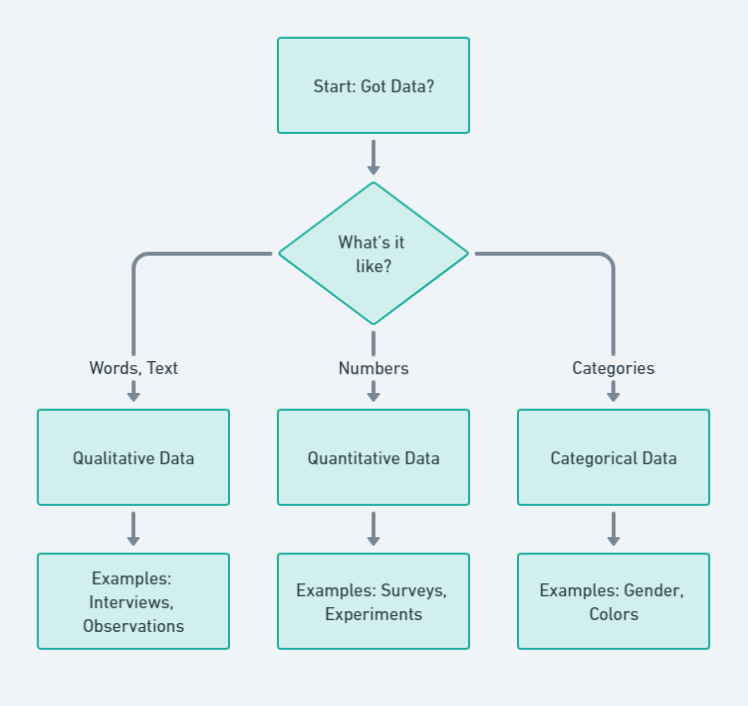
Data Analysis in Qualitative Research
Hey, future business moguls! So you’ve gathered a bunch of qualitative data—customer reviews, employee feedback, and maybe even some brand mentions on social media. Now what? Let’s dig in!
How It Works
Think of qualitative research like being the CEO of your own startup. You’ve got all this raw material (your data), and you need to turn it into a finished product (insights). You’ll sift through the data, looking for trends, themes, or even contradictions that can help you make informed business decisions.
Methods Used
There are several methods to analyze qualitative data, each with its own unique approach. Let’s get into the details:
Content Analysis 📄
This is your bread and butter for analyzing text-based data. You’ll categorize your data into themes and then analyze those themes.
Examples:
- Customer Reviews: Analyzing what customers are saying about your product on Amazon.
- Employee Surveys: Understanding the common themes in employee satisfaction or dissatisfaction.
- Social Media Mentions: What are people saying about your brand on Twitter?
- Email Feedback: Categorizing the types of complaints or praises received via customer service emails.
- Website Comments: What are visitors saying in the comments section of your blog posts?
Narrative Analysis
This method helps you understand the structure and content of stories or experiences.
Examples:
- Customer Testimonials: Understanding the customer journey through success stories.
- Case Studies: Analyzing the narrative of how your product solved a problem.
- Sales Pitches: What stories are most effective in closing deals?
- Employee Exit Interviews: Understanding the stories behind why employees leave.
- Investor Presentations: Analyzing the narrative structure of successful pitches.
Discourse Analysis
Here, you’re looking at the context—how language shapes and is shaped by social norms and attitudes.
Examples:
- Team Meetings: How does language affect team dynamics?
- Customer Service Calls: What language leads to successful conflict resolution?
- Marketing Materials: How does language affect customer perception?
- Public Relations Statements: Analyzing the discourse in crisis management.
- Negotiation Transcripts: What language leads to more favorable business deals?
Grounded Theory
Start with zero assumptions and let the data guide you to a theory.
Examples:
- Market Research: Understanding consumer behavior from scratch.
- Product Development: Building a new feature based on raw user feedback.
- Competitive Analysis: Understanding your market position without preconceived notions.
- Organizational Culture: Building a company culture based on employee feedback.
- Business Strategy: Formulating a new business model based on various data points.
Let’s Compare!
Method | Ease of Use | Required Tools | Data Types | Best For |
---|---|---|---|---|
Content Analysis | Easy | Text Editor | Text, Images | Media Studies |
Narrative Analysis | Moderate | Text Editor | Stories, Interviews | Psychology |
Discourse Analysis | Hard | Specialized Software | Conversations, Text | Sociology |
Grounded Theory | Moderate | Specialized Software | Observations, Interviews | Social Sciences |
Each method has its own pros and cons, so pick the one that aligns best with your business goals.
Finding Patterns in Qualitative Data
Hey there, future data wizards! So you’ve got this mountain of qualitative data, and you’re wondering how to find those hidden gems—those patterns that will give you the “Aha!” moments. Let’s get into it!
Techniques for Finding Patterns
Finding patterns in qualitative data is like going on a treasure hunt. You have a map (your data), and you’re looking for the X that marks the spot (patterns). Here are some techniques to help you find that treasure:
Word-Based Methods
This is all about looking for specific words or phrases that appear frequently in your data.
Examples:
- Customer Reviews: Identifying common words like “excellent service” or “poor quality.”
- Employee Feedback: Spotting recurring words like “overworked” or “great culture.”
- Market Research: Noticing frequent mentions of “affordable” or “luxury.”
- Social Media Monitoring: Picking up on trending hashtags related to your brand.
- User Interviews: Highlighting repeated terms like “easy to use” or “complicated.”
Keyword Context
Here, you’re not just looking at the words themselves but also the context in which they appear.
Examples:
- Customer Emails: Understanding the issues surrounding words like “refund” or “defective.”
- Sales Calls: Noticing the context in which “discount” or “contract” are discussed.
- Focus Groups: Observing the circumstances when participants mention “trust” or “safety.”
- Product Reviews: Analyzing the context around words like “innovative” or “outdated.”
- Investor Relations: Understanding the context in which terms like “ROI” or “risk” are used.
Variable Partitioning
This involves dividing your data into smaller parts based on variables like age, location, or any other factor that might affect the pattern.
Examples:
- Customer Segmentation: Finding patterns in feedback based on age groups.
- Regional Sales: Partitioning sales data by geographic location.
- Employee Satisfaction: Dividing data based on departments or job roles.
- Product Usage: Segmenting user data based on the frequency of product use.
- Campaign Effectiveness: Partitioning marketing data based on the advertising platform used.
Let’s Compare!
Method | Frequency of Use |
---|---|
Word-Based Methods | 45% |
Keyword Context | 30% |
Variable Partitioning | 25% |
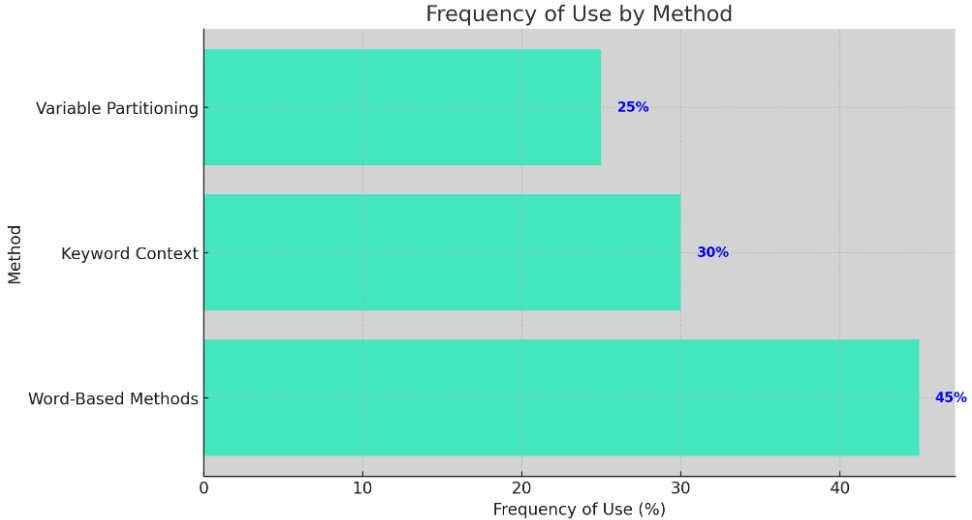
Note: The percentages represent the frequency with which these methods are commonly used in business settings.
Data Analysis in Quantitative Research
Hey there, future number crunchers! So you’ve got a bunch of numbers and you’re ready to dive into the world of quantitative research. But wait! Before you start running those fancy algorithms, there’s some groundwork to be done. Let’s break it down!
Preparing Data for Analysis
Think of this stage as the prep work you do before cooking a delicious meal. You’ve got to chop the veggies, marinate the meat, and set the table before you can feast. Similarly, your data needs some prepping too!
Data Validation
First things first, you’ve got to make sure your data is legit. This means checking for any errors or inconsistencies that could mess up your analysis.
Examples:
- Survey Responses: Make sure there are no contradictory answers.
- Sales Data: Confirm that all transactions are accurately recorded.
- Website Analytics: Verifying that the tracking codes are correctly implemented.
- Customer Feedback: Ensuring that the feedback is from actual customers and not bots.
- Inventory Levels: Double-check that the numbers match the physical stock.
Data Editing
Once you’ve validated your data, the next step is to clean it up. This involves correcting or removing any inaccuracies.
Examples:
- Duplicate Entries: Removing any repeated records in your CRM.
- Outliers: Identifying and handling data points that are way off the norm.
- Incomplete Records: Filling in missing values or deciding how to handle them.
- Date Formats: Make sure all dates are in a consistent format.
- Currency Conversion: If you’re dealing with international sales, convert all figures to a single currency.
Data Coding
This is the final step before you can start your analysis. Data coding involves converting your cleaned-up data into a format that can be easily analyzed. Think of it as translating your data into a language that your analysis tools can understand. 🗨️
Examples:
- Customer Ratings: Converting text-based ratings like “Excellent” or “Poor” into numerical values.
- Product Categories: Assigning codes to different types of products.
- Employee IDs: Use unique identifiers for each employee in your analysis.
- Sales Channels: Coding different sales channels like “Online,” “In-Store,” or “Third-Party.”
- Seasonal Data: Coding data based on seasons like “Q1,” “Summer,” or “Holiday Season.”
Steps for Preparing Data for Quantitative Analysis
- Collect Raw Data: Gather all the data you need for your research.
- Data Validation: Check for errors or inconsistencies.
- Data Editing: Clean up any inaccuracies.
- Data Coding: Convert data into an analyzable format.
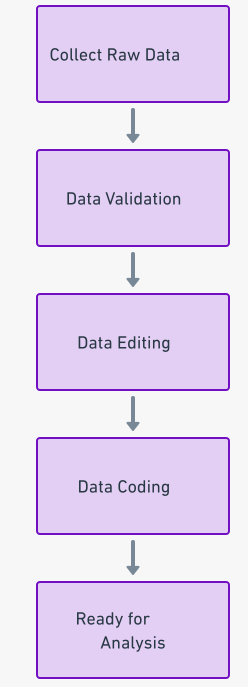
Methods Used for Data Analysis in Quantitative Research
Alright, data enthusiasts! Now that your data is all prepped and ready to go, it’s time to dive into the actual analysis. But wait, there are different ways to go about this, and each has its own special sauce. Let’s explore!
Descriptive Statistics
This is like taking a selfie of your data. You’re capturing what it looks like at a specific moment in time. Descriptive statistics help you summarize and understand the basic features of your data.
Examples:
- Average Sales: Calculating the mean sales per month or quarter.
- Customer Age Range: Finding the minimum and maximum ages of your customer base.
- Employee Satisfaction: Using the mode to find the most common satisfaction rating among employees.
- Product Ratings: Calculating the median rating for your products.
- Website Traffic: Summarizing daily visits to your website.
Inferential Statistics
Now, this is where the magic happens! Inferential statistics allow you to make predictions or inferences about a larger population based on a sample.
Examples:
- Market Trends: Predicting future sales based on past data.
- Customer Behavior: Making inferences about customer preferences.
- Employee Retention: Predicting turnover rates based on current data.
- Product Success: Estimating how well a new product will do in the market.
- Advertising Effectiveness: Assessing the impact of a marketing campaign on sales.
Key Statistical Methods and When to Use Them
Method | What It Does | When to Use It |
---|---|---|
Mean | Average of all values | Summarizing a typical data point |
Median | Middle value in a sorted list | When data has outliers |
Mode | Most frequently occurring value | Identifying common trends |
Standard Deviation | Measures data variability | Understanding data spread |
Correlation | Measures relationship between variables | Identifying patterns between variables |
T-Test | Compares means of two groups | Testing differences between groups |
Chi-Square Test | Tests relationships between categories | Understanding associations in categorical data |
Regression Analysis | Predicts the outcome of a variable | Making predictions based on data |
ANOVA (Analysis of Variance) | Compares means among multiple groups | When comparing more than two groups |
Descriptive Statistics
Alright, let’s dig deeper into the world of descriptive statistics! Descriptive stats help you summarize and understand your data, and there are several types of measures you can use to do this. Let’s break it down:
Measures of Frequency
This is all about counting how often something happens. For example, how many times a specific product was sold last month?
Examples:
- Daily Sales: Counting the number of sales each day.
- Customer Visits: Tracking the frequency of customer visits to a store.
- Social Media Engagement: Measuring the number of likes, shares, and comments.
- Inventory Turnover: Counting how often inventory is sold and replaced.
- Employee Absenteeism: Tracking the frequency of employee absences.
Measures of Central Tendency
This helps you find the “middle” or “average” of your data.
Examples:
- Average Revenue: Calculating the mean revenue per customer.
- Median Age of Employees: Finding the middle age in your workforce.
- Mode of Customer Ratings: Identifying the most common rating given by customers.
- Average Order Value: Calculating the mean value of all orders.
- Median Time on Site: Finding the middle amount of time users spend on your website.
Measures of Dispersion
This tells you how spread out your data is.
Examples:
- Price Range: The range between the cheapest and most expensive products.
- Employee Salary Variance: How much salaries vary within a department.
- Customer Lifetime Value Variability: The spread in the lifetime value of different customer segments.
- Product Rating Variability: The range of product ratings.
- Sales Fluctuations: The variance in daily or monthly sales.
Measures of Position
This helps you understand where a particular data point stands in relation to others.
Examples:
- Top 10% of Salespeople: Identifying the highest-performing sales reps.
- Lowest-Grossing Products: Finding the products that are in the bottom 10% of sales.
- Customer Quartiles: Dividing customers into quartiles based on spending.
- Employee Performance Percentiles: Ranking employees based on performance metrics.
- Website Traffic Deciles: Dividing website traffic into deciles to analyze user behavior.
Inferential Statistics
Hey there, future data wizards! So you’ve got the basics of descriptive statistics down, and now you’re ready to level up. Enter inferential statistics! This is where you get to make educated guesses (or, you know, “inferences”) about a whole population based on a sample. Let’s dive in!
Estimating Parameters
In inferential statistics, parameters are the key characteristics of a population. Estimating them helps you make educated guesses about the entire group based on your sample data.
Business Examples:
- Market Share: Estimating your company’s market share based on a sample survey.
- Customer Lifetime Value: Using sample data to estimate the average lifetime value of all customers.
- Employee Satisfaction: Estimating the overall job satisfaction level within the company based on a sample survey.
- Product Failure Rate: Using sample testing to estimate how often a product might fail in real-world conditions.
- Conversion Rate: Estimating the percentage of website visitors who will make a purchase.
Hypothesis Testing
This is the scientific part of inferential statistics. You start with a hypothesis (an educated guess) and then use your data to see if it holds up.
Business Examples:
- A/B Testing: Testing two different website designs to see which one results in more sales.
- Product Pricing: Testing different price points to determine which maximizes revenue.
- Customer Segmentation: Testing whether different customer groups have different spending habits.
- Employee Benefits: Testing the impact of new employee benefits on job satisfaction.
- Advertising Channels: Testing the effectiveness of different advertising channels on customer acquisition.
Examples of Research Questions and Appropriate Methods
Research Question | Appropriate Method |
---|---|
“Does changing the website layout increase sales?” | A/B Testing |
“Is there a difference in spending between age groups?” | ANOVA (Analysis of Variance) |
“Does employee satisfaction affect productivity?” | Correlation Analysis |
“Will a price increase result in lower sales?” | Regression Analysis |
“Do customers prefer online shopping over in-store?” | Chi-Square Test |
Tools and Software for Data Analysis
Hey there, tech-savvy peeps! So you’ve got all this data and you’re ready to dive into analysis. But wait, you’re gonna need the right tools for the job! Whether you’re into qualitative or quantitative research, there’s a software out there that’s just perfect for you. Let’s explore!
Quantitative Research Tools
For those of you who love numbers and hard data, quantitative research is your playground. Here are some popular tools and software you can use:
Business Examples:
- Surveys/Questionnaires: SPSS is a go-to for analyzing survey data. It’s paid, but worth it!
- Interviews: Stata is another paid option that’s great for crunching numbers from interviews.
- Observations: SAS is a powerful tool for observational data, but it comes with a price tag.
- Experiments: R is a free software that’s perfect for experimental data.
- Controlled Observations: MATLAB is a paid tool that excels in this area.
Features and Costs of Different Tools for Quantitative Research
Quantitative Research Tools | Software for Data Analysis | Paid/Free |
---|---|---|
Surveys/Questionnaires | SPSS | Paid |
Interviews | Stata | Paid |
Observations | SAS | Paid |
Experiments | R | Free |
Controlled Observations | MATLAB | Paid |
Longitudinal Studies | Excel | Paid (Part of Microsoft Office Suite) |
Polls | Google Analytics | Free |
Telephone Interviews | JMP | Paid |
Face-to-Face Interviews | Python | Free |
Physical Tests |
Qualitative Research Tools
For those who are more into understanding human behavior, emotions, and experiences, qualitative research is where it’s at. Here are some popular tools and software you can use, along with examples of when you might want to use them:
Business Examples:
- ATLAS.ti: Perfect for analyzing customer feedback and reviews.
- Dedoose: Ideal for sorting through focus group discussions to identify common themes.
- NVivo: Great for coding and analyzing textual data from interviews.
- Cauliflower: Useful for sentiment analysis in social media comments.
- Marvin: Excellent for analyzing video and audio data from customer interactions.
Features and Costs of Different Tools for Qualitative Research
Tool/Software | Paid/Free |
---|---|
ATLAS.ti | Paid |
Dedoose | Paid |
NVivo | Paid |
Cauliflower | Paid |
Marvin | Paid |
Dovetail | Paid |
Qualdesk | Paid |
Sticktail | Paid |
EnjoyHQ | Paid |
Condens | Paid |
Talebook | Paid |
MAXQDA | Paid |
HyperRESEARCH | Paid |
Quirkos | Paid |
This pie chart serves as a checklist that researchers can refer to for key considerations like ethical considerations, data quality, and more.
So, are you all set to dive into data analysis with all these considerations in mind? Let’s go!
Key Considerations in Research Data Analysis Checklist
Ethical Considerations
- [ ] Informed Consent: Get permission before using someone’s data.
- [ ] Data Anonymization: Remove personal identifiers from your data.
- [ ] Transparency: Be open about your methods and intentions.
- [ ] Fairness: Ensure your research doesn’t discriminate.
- [ ] Data Integrity: Don’t manipulate data to fit your narrative.
Data Quality
- [ ] Data Cleaning: Remove outliers or errors.
- [ ] Data Verification: Double-check data sources for reliability.
- [ ] Data Relevance: Ensure data is relevant to your research question.
- [ ] Data Completeness: Include all necessary data points.
- [ ] Data Timeliness: Use the most recent data for time-sensitive research.
Data Security
- [ ] Secure Storage: Use encrypted storage solutions.
- [ ] Secure Transfer: Use secure methods like SSL for data transfer.
- [ ] Access Control: Limit who can access the data.
- [ ] Regular Audits: Conduct security audits to identify vulnerabilities.
- [ ] Data Backup: Regularly back up data to a secure location.
Data Privacy
- [ ] GDPR Compliance: Comply with data protection laws.
- [ ] User Consent: Don’t collect more data than necessary.
- [ ] Data Minimization: Only collect required data.
- [ ] Privacy Policy: Clearly outline your privacy policy.
- [ ] Data Retention: Don’t keep data longer than necessary.
Data Interpretation
- [ ] Bias Avoidance: Be aware of biases that could affect interpretation.
- [ ] Contextual Analysis: Interpret data within its context.
- [ ] Peer Review: Have findings reviewed by experts.
- [ ] Sensitivity Analysis: Check how different assumptions affect conclusions.
- [ ] Transparency: Clearly state any limitations in your data or methodology.
Conclusion
Wow, what a journey, right? We’ve covered everything from the ABCs of data analysis to the ethical considerations you need to keep in mind.
Quick Recap
- Importance: Data analysis is the heartbeat of research. It helps you make sense of all the data you’ve collected and turn it into actionable insights.
- Types: Whether you’re into numbers with quantitative data or more about feelings and experiences with qualitative data, there’s a method out there for you.
- Methods: From content analysis in qualitative research to descriptive and inferential statistics in quantitative research, the methods are as diverse as they are powerful.
Ready to Level Up?
If you’re as excited about data analysis as we are, why stop here? Take your skills to the next level with my course on data analysis for research. Trust me, it’s a game-changer!
Take My Course on Data Analysis for Research
So, are you ready to become a data analysis superstar? Let’s make research more meaningful, one data point at a time!
Catch you in the course!